An overview of Optimization and learning algorithms for energy management in smart homes
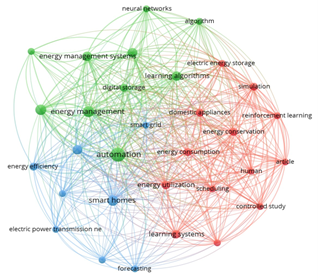
Downloads
Smart home electric energy management systems are designed to optimize energy efficiency, reduce waste, and promote sustainability. This paper aims to conduct a literature review on optimization and learning algorithms for energy management in smart homes. The research methodology involves retrieving data from the Scopus database and visualizing the information through VOSviewer. The results of the literature reviewed encompass a wide range of strategies and algorithms aimed at optimizing energy management in smart homes. A key noticeable aspect is the diverse application of advanced optimization techniques and machine learning algorithms to enhance energy efficiency, reduce costs, and promote sustainability. The study contributes by providing an in-depth analysis of optimization algorithms for energy cost reduction, evaluating and categorizing learning algorithms, and identifying best practices for optimizing energy consumption in smart homes.
Neogy T.K, Bynagari N.B, “Gradient Descent is a Technique for Learning to Learn”, Asian of Journal Humanity, Art and Literature, vol.5, 2018, pp.2311-8636.
Ke Tang1, and Xin Yao,” Learn to optimize—a brief overview”, Oxford University Press, vol. 11, 2024, pp. 132.
Frank E. Curtis, Katya Scheinberg, “Optimization Methods for Supervised Machine Learning: From Linear Models to Deep Learning”, vol. 1, 2017, pp. 1706-10207.
Nikhil G. Kurup, Dr. K. S. Vijula Grace,” Optimisation Algorithms for Deep Learning Method: A Review with a Focus on Financial Applications”, AIJR Publisher, vol.10, 2023, pp.289-296.
Khan, W. A., Chung, S. H., Awan, M. U., & Wen, X, “Machine learning facilitated business intelligence (Part II): Neural networks optimization techniques and applications”, Emerald Publishing Limited, vol.120, 2019, pp. 128–163.
Eric Breimer, Mark Goldberg, David Hollinger, and Darren Lim, “Discovering Optimization Algorithms Through Automated Learning”, Computer Science Department, Rensselaer Polytechnic Institute, 2005.
Rahul Paul and Kedar Nath Das, “Trends of Optimization Algorithms from Supervised Learning Perspective”, Journal of Computational and Cognitive Engineering, vol. 00, 2023, pp 00.
Neeraj kumar, Kalyana Sundaram, Reena R and Madhumathi,” OPTIMIZING ENERGY CONSUMPTION IN SMART HOMES USING MACHINE LEARNING TECHNIQUES”, E3S Web of Conferences, vol.02, 2023, pp.2.
Wafa Shafqat, Kyu-Tae Lee, and Do-Hyeun Kim, “A Comprehensive Predictive-Learning Framework for Optimal Scheduling and Control of Smart Home Appliances Based on User and Appliance Classification”, Republic of Korea, vol.23, 2022, pp.127.
Senthil Prabu Ramalingam and Prabhakar Karthikeyan Shanmugam,” Investigation on Optimization Algorithms for Smart Home Energy Management with Different Electricity Pricing”, International Journal of Electrical and Electronic Engineering & Telecommunications, vol. 11, 2022, pp. 6.
Tesfahun Molla, Baseem Khan, Pawan Singh,” A comprehensive analysis of smart home energy management system optimization techniques”, Journal of Autonomous Intelligence, vol.1, 2018, pp.1.
Abdul Salam Shah, Haidawati Nasir, Muhammad Fayaz, Adidah Lajis and Asadullah Shah,” A Review on Energy Consumption Optimization Techniques in IoT Based Smart Building Environments”, information, vol.10, 2019, pp.108.
Isaías Gomes, Karol Bot, Maria Graça Ruano and António Ruano,” Recent Techniques Used in Home Energy Management Systems: A Review”, Energies, vol. 15, 2022, pp. 2866.
Sonja Kallio and Monica Siroux,” A Review – Home Renewable Energy Management Systems in Smart Grids”, Earth and Environmental Science, vol. 10, 2022, pp.1755-1315.
Yuankun Liu, Dongxia Zhang, and Hoay Beng Gooi,” Optimization Strategy Based on Deep Reinforcement Learning for Home Energy Management”, JOURNAL OF POWER AND ENERGY SYSTEMS, vol.6, 2020, pp. 3.
Dai H, Ningfan Li, Yuhan Wang, Xinrui Zhao,” The analysis of three main investment criteria: NPV IRR and payback period, ” atlantis press international B.V, vol.211,4, 185-186, 2022.
Calculating the return investment for energy, silverman-associates, planning, advice, accountability, 10 December 2024. [Online] Available: https://silverman-associates.com/blog/calculating-the-return-on-investment-for-energy-efficient-home-upgrades, accessed 22 January 2025.
B Miriam, F Joshua, Yue Nicole Wu, C Zamawe, Lan Hamilton, John Eyers,”Residential energy efficiency intervention: A metal analysis of effectiveness studies,” IEEE Trans.campbell systematic reviews, vol.17, pp.e1206, doi:10.1002/c12.1206.
Khan N, Z Shahid, MM Alan, sajak A.A.B, M.S Maziliham, Khan T.A, Rizvi S.S.A,”Energy management systems using smart grids: an exhaustive parametric comprehensive analysis of existing trends, significance, opportunities, and challenges,” IEEE Trans Hindawi, vol.2022, pp. 1155, 17 may 2022, doi: 10.1155/2022/3358795.
Zhang M.; Zhang D.; Xie T.,”Balancing urban energy considering economic growth and environmental sustainability through integration of renewable energy”,Sustainable Cities and Society,vol. 101, 2024, pp. 105178.
Mahjoub S.; Labdai S.; Chrifi-Alaoui L.; Marhic B.; Delahoche L.,” Short-Term Occupancy Forecasting for a Smart Home Using Optimized Weight Updates Based on GA and PSO Algorithms for an LSTM Network”, Energies, vol. 16, 2023, pp. 1641.
Vasudevan N.; Venkatraman V.; Ramkumar A.; Sheela A.,” Real-time day ahead energy management for smart home using machine learning algorithm”, Journal of Intelligent and Fuzzy Systems, vol. 41, 2021, pp. 5665-5676.
Meng H.; Feng S.; Li C.,” An integrated system of energy generation, storages, and appliances consumption based on machine learning techniques and internet of things”, Journal of Energy Storage, vol. 87, 2024, pp. 111380.
Franco P.; Martinez J.M.; Kim Y.-C.; Ahmed M.A.,” A framework for iot based appliance recognition in smart homes”, IEEE Access, vol. 9, 2021, pp. 133940-133960.
Cabral T.W.; Lemes D.A.M.; Fraidenraich G.; Neto F.B.; De Lima E.R.; Meloni L.G.P.,” High-Reliability Load Recognition in Home Energy Management Systems”, IEEE Access, vol. 11, 2023, pp. 31244-31261.
Keerthisinghe C.; Chapman A.C.; Verbič G.,” Energy Management of PV-Storage Systems: Policy Approximations Using Machine Learning”, IEEE Transactions on Industrial Informatics, vol. 15, 2019, pp. 257-265.
Tundis A.; Faizan A.; Mühlhäuser M.,” A feature-based model for the identification of electrical devices in smart environments”, Sensors (Switzerland), vol. 19, 2019, pp. 2611.
Lee S.; Choi D.-H.,” Reinforcement learning-based energy management of smart home with rooftop solar photovoltaic system, energy storage system, and home appliances”, Sensors (Switzerland), vol. 19, 2019, pp. 3937.
Tai C.-S.; Hong J.-H.; Hong D.-Y.; Fu L.-C.,” A real-time demand-side management system considering user preference with adaptive deep Q learning in home area network”, Sustainable Energy, Grids and Networks, vol. 29, 2022, pp. 100572.
Khan M.; Seo J.; Kim D.,” Towards energy efficient home automation: A deep learning approach”, Sensors (Switzerland), vol. 20, 2020, pp. 1-18.
Ghosh S.; Chatterjee D.,” Artificial Bee Colony Optimization Based Non-Intrusive Appliances Load Monitoring Technique in a Smart Home”, IEEE Transactions on Consumer Electronics, vol. 67, 2021, pp. 77-86.
Lee S.; Choi D. H. ” Energy management of smart home with home appliances, energy storage system and electric vehicle: A hierarchical deep reinforcement learning approach”, Sensors (Switzerland), vol. 20, 2020, pp. 2157.
Leonori S.; Martino A.; Frattale Mascioli F.M.; Rizzi A.,” Microgrid Energy Management Systems Design by Computational Intelligence Techniques”, Applied Energy, vol. 277, 2020, pp. 115524.